Application of the EEMD Algorithm for the Monitoring of the Cutting Tool wear
Keywords:
Algorithm , EMD, EEMD, Monitoring, Cutting tool, WearAbstract
In this work, it is a question of a monitoring of cutting tool wear in mechanical turning. This monitoring is carried out in three phases which correspond to the life of the tool. To achieve this objective of improving the monitoring of the cutting tool, we have proposed the EEMD processing algorithm which decomposes a large signal into small signals (IMFs) by comparing it to the EMD algorithm, which is an algorithm used in the analysis of non-linear and non-stationary signals. The phenomenon of mode mixing is one of the major drawbacks of EMD. The EEMD eliminates the mode mixing effect. The EEMD principle is to add extra white noise to the signal with a certain number of tries.
Downloads
References
L. Dan and J. Mathiew , "Tool Wear Failure Monitoring Technique for Turning", Int.J Mach. Tools Manuf ., vol. 30, p. 579, 1990.
M. Nouari , "Modeling wear by diffusion of cutting tools in high-speed machining", doctoral thesis from the University of Metz, 2000.
A.Devillez , S.Lesko , and W.Mozer , "Cutting tool crater wear measurement with white light interferometry", WEAR, vol. 256, p. 56-65, 2004.
NE Huang, Z. Shen, SR Long, MC Wu, HH Shin, Q. Zheng, NC Yen, CC Tung, and HH Liu, “The empirical mode decomposition and the Hilbert spectrum for nonlinear and non-stationary time series analysis,” Proc. R. Soc. London . A, Math. Phys. Science, vol. 454, no. 1971, p. 903–995, March 1998.
Huang, NE, & Wu, ZH (2008). A Review of the Hilbert-Huang Transformed Form: Method and its Applications to Geophysical Studies Revues de Géophysique, 46(2), 1–23
Mohammad, SH, Siamak , EK, & Mohammad, SS (2018). Quantitative diagnosis for bearing faults by improving ensemble empirical mode decomposition. ISA Transactions, 83, 261–275.
Hikmat , H., Maha , A., & Enas , A. (2018). Automated identification for autism severity level: EEG analysis using empirical mode decomposition and second order difference plot. Behavioral Brain Research, 362, 240–248.
Qin, Y., Qiao , LH, Wang, QF, Ren, XZ, & Zhu, CH (2018). Bidimensional empirical mode decomposition method for image processing in sensing system. Computers & Electrical Engineering, 68, 215–224. March 2018
NE Huang, Z. Shen, SR Long, MC Wu, HH Shin, Q. Zheng, NC Yen, CC Tung, and HH Liu, “The empirical mode decomposition and the Hilbert spectrum for nonlinear and non-stationary time series analysis,” Proc. R. Soc. London . A, Math. Phys. Science, vol. 454, no. 1971, p. 903–995, March 1998.
BOVIC KILUNDU. "Detection and vibration diagnosis of mechanical degradation: contribution of data minig " PhD Thesis Faculty of Polytechnique of Mons. 2008
Zhang J., Yan R., Gao RX, Feng Z., Performance enhancement of ensemble empirical mode decomposition, Mechanical Systems and Signal Processing., 24 (7): 2104-2123, 2010.
Wu Z., Huang NE, Ensemble empirical mode decomposition: a noise- assisted data analysis method. Adv. Adapt. Data Anal., 1 (1): 1–41, 2009.
Rilling, G., & Flandrin, P. (2008). One or two frequencies? The empirical mode decomposition answers. IEEE Transactions on Signal Processing, 56(1), 85-95.
Torres M.E., Colominas M.A., Schlotthauer G., Flandrin P. (2011) A complete ensemble empirical mode decomposition with adaptive noise, in: Proceedings of the 36th IEEE International Conference on Acoustics, Speech, and Signal Processing (ICASSP), IEEE, pp. 4144–4147.
J.C. Cexus, "Analysis of non-stationary signals by Huang transform, Teager-Kaiser operator and Huang-Teager transform (THT)" PhD thesis, University of Rennes1, 2005.
Salisbury J. I., Sun Y., Rapid screening test for sleep apnea using a nonlinear and non stationary signal processing technique. Med. Eng. Phys., 29 (3): 336–343, 2007.
G. Rilling, "Empirical Modal Decomposition - Contributions to the theory, algorithm and performance analysis" PhD thesis, University Lyon-Ecole Normale Supérieure de Lyon, 2007.
Huang, N. E., & Wu, Z. (2008). A review on Hilbert-Huang transform: method and its applications to geophysical studies. Reviews of Geophysics, 46(2), RG2006.
Numes J. C., Deléchelle E., Empirical mode decomposition: Application on signal and
image processing. Advances in Adaptive Data Analysis., 1(1):125–175, 2009.
Wu, Z., & Huang, N. E. (2009). Ensemble empirical mode decomposition: a noise-assisted data analysis method. Advances in Adaptive Data Analysis, 1(1), 1-41.
Flandrin P., Rilling G., et Gonçalvès P., Empirical mode decomposition as a filter bank. IEEE Signal Processing Letters., 11(2):112–114, 2004.
Rilling G., Flandrin P., et Goncalvès P., On empirical mode decomposition and its algorithms. In IEEE-EURASIP, Workshop on Non linear Signal and Image Processing, NSIP ’03, Grado (I), juin 2003.
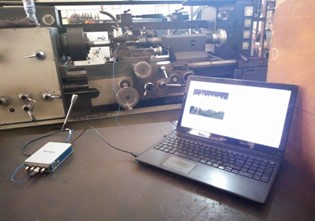
Downloads
Published
How to Cite
Issue
Section
ARK
License
Copyright (c) 2023 claude mukaz

This work is licensed under a Creative Commons Attribution 4.0 International License.
Copyright on any article in the International Journal of Engineering and Applied Physics is retained by the author(s) under the Creative Commons license, which permits unrestricted use, distribution, and reproduction provided the original work is properly cited.
License agreement
Authors grant IJEAP a license to publish the article and identify IJEAP as the original publisher.
Authors also grant any third party the right to use, distribute and reproduce the article in any medium, provided the original work is properly cited.
Most read articles by the same author(s)
- claude mukaz, Moise NGOYI KASANJI, Application of the EEMD and CEEMDAN algorithm for non- linear signal processing , International Journal of Engineering and Applied Physics: Vol. 3 No. 2: May 2023